roc_auc_score#
- sklearn.metrics.roc_auc_score(y_true, y_score, *, average='macro', sample_weight=None, max_fpr=None, multi_class='raise', labels=None)[source]#
Compute Area Under the Receiver Operating Characteristic Curve (ROC AUC) from prediction scores.
Note: this implementation can be used with binary, multiclass and multilabel classification, but some restrictions apply (see Parameters).
Read more in the User Guide.
- Parameters:
- y_truearray-like of shape (n_samples,) or (n_samples, n_classes)
True labels or binary label indicators. The binary and multiclass cases expect labels with shape (n_samples,) while the multilabel case expects binary label indicators with shape (n_samples, n_classes).
- y_scorearray-like of shape (n_samples,) or (n_samples, n_classes)
Target scores.
In the binary case, it corresponds to an array of shape
(n_samples,)
. Both probability estimates and non-thresholded decision values can be provided. The probability estimates correspond to the probability of the class with the greater label, i.e.estimator.classes_[1]
and thusestimator.predict_proba(X, y)[:, 1]
. The decision values corresponds to the output ofestimator.decision_function(X, y)
. See more information in the User guide;In the multiclass case, it corresponds to an array of shape
(n_samples, n_classes)
of probability estimates provided by thepredict_proba
method. The probability estimates must sum to 1 across the possible classes. In addition, the order of the class scores must correspond to the order oflabels
, if provided, or else to the numerical or lexicographical order of the labels iny_true
. See more information in the User guide;In the multilabel case, it corresponds to an array of shape
(n_samples, n_classes)
. Probability estimates are provided by thepredict_proba
method and the non-thresholded decision values by thedecision_function
method. The probability estimates correspond to the probability of the class with the greater label for each output of the classifier. See more information in the User guide.
- average{‘micro’, ‘macro’, ‘samples’, ‘weighted’} or None, default=’macro’
If
None
, the scores for each class are returned. Otherwise, this determines the type of averaging performed on the data. Note: multiclass ROC AUC currently only handles the ‘macro’ and ‘weighted’ averages. For multiclass targets,average=None
is only implemented formulti_class='ovr'
andaverage='micro'
is only implemented formulti_class='ovr'
.'micro'
:Calculate metrics globally by considering each element of the label indicator matrix as a label.
'macro'
:Calculate metrics for each label, and find their unweighted mean. This does not take label imbalance into account.
'weighted'
:Calculate metrics for each label, and find their average, weighted by support (the number of true instances for each label).
'samples'
:Calculate metrics for each instance, and find their average.
Will be ignored when
y_true
is binary.- sample_weightarray-like of shape (n_samples,), default=None
Sample weights.
- max_fprfloat > 0 and <= 1, default=None
If not
None
, the standardized partial AUC [2] over the range [0, max_fpr] is returned. For the multiclass case,max_fpr
, should be either equal toNone
or1.0
as AUC ROC partial computation currently is not supported for multiclass.- multi_class{‘raise’, ‘ovr’, ‘ovo’}, default=’raise’
Only used for multiclass targets. Determines the type of configuration to use. The default value raises an error, so either
'ovr'
or'ovo'
must be passed explicitly.'ovr'
:Stands for One-vs-rest. Computes the AUC of each class against the rest [3] [4]. This treats the multiclass case in the same way as the multilabel case. Sensitive to class imbalance even when
average == 'macro'
, because class imbalance affects the composition of each of the ‘rest’ groupings.'ovo'
:Stands for One-vs-one. Computes the average AUC of all possible pairwise combinations of classes [5]. Insensitive to class imbalance when
average == 'macro'
.
- labelsarray-like of shape (n_classes,), default=None
Only used for multiclass targets. List of labels that index the classes in
y_score
. IfNone
, the numerical or lexicographical order of the labels iny_true
is used.
- Returns:
- aucfloat
Area Under the Curve score.
See also
average_precision_score
Area under the precision-recall curve.
roc_curve
Compute Receiver operating characteristic (ROC) curve.
RocCurveDisplay.from_estimator
Plot Receiver Operating Characteristic (ROC) curve given an estimator and some data.
RocCurveDisplay.from_predictions
Plot Receiver Operating Characteristic (ROC) curve given the true and predicted values.
Notes
The Gini Coefficient is a summary measure of the ranking ability of binary classifiers. It is expressed using the area under of the ROC as follows:
G = 2 * AUC - 1
Where G is the Gini coefficient and AUC is the ROC-AUC score. This normalisation will ensure that random guessing will yield a score of 0 in expectation, and it is upper bounded by 1.
References
[3]Provost, F., Domingos, P. (2000). Well-trained PETs: Improving probability estimation trees (Section 6.2), CeDER Working Paper #IS-00-04, Stern School of Business, New York University.
[4]Fawcett, T. (2006). An introduction to ROC analysis. Pattern Recognition Letters, 27(8), 861-874.
[5]Examples
Binary case:
>>> from sklearn.datasets import load_breast_cancer >>> from sklearn.linear_model import LogisticRegression >>> from sklearn.metrics import roc_auc_score >>> X, y = load_breast_cancer(return_X_y=True) >>> clf = LogisticRegression(solver="liblinear", random_state=0).fit(X, y) >>> roc_auc_score(y, clf.predict_proba(X)[:, 1]) 0.99... >>> roc_auc_score(y, clf.decision_function(X)) 0.99...
Multiclass case:
>>> from sklearn.datasets import load_iris >>> X, y = load_iris(return_X_y=True) >>> clf = LogisticRegression(solver="liblinear").fit(X, y) >>> roc_auc_score(y, clf.predict_proba(X), multi_class='ovr') 0.99...
Multilabel case:
>>> import numpy as np >>> from sklearn.datasets import make_multilabel_classification >>> from sklearn.multioutput import MultiOutputClassifier >>> X, y = make_multilabel_classification(random_state=0) >>> clf = MultiOutputClassifier(clf).fit(X, y) >>> # get a list of n_output containing probability arrays of shape >>> # (n_samples, n_classes) >>> y_pred = clf.predict_proba(X) >>> # extract the positive columns for each output >>> y_pred = np.transpose([pred[:, 1] for pred in y_pred]) >>> roc_auc_score(y, y_pred, average=None) array([0.82..., 0.86..., 0.94..., 0.85... , 0.94...]) >>> from sklearn.linear_model import RidgeClassifierCV >>> clf = RidgeClassifierCV().fit(X, y) >>> roc_auc_score(y, clf.decision_function(X), average=None) array([0.81..., 0.84... , 0.93..., 0.87..., 0.94...])
Gallery examples#
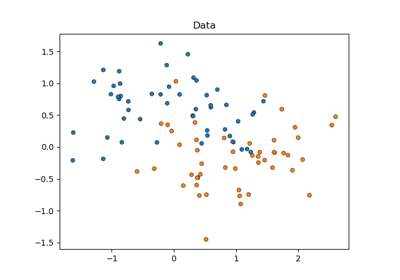
Statistical comparison of models using grid search
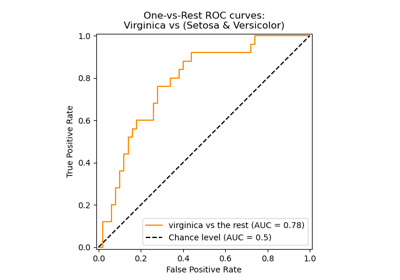
Multiclass Receiver Operating Characteristic (ROC)