Note
Go to the end to download the full example code. or to run this example in your browser via JupyterLite or Binder
Nearest Neighbors regression#
Demonstrate the resolution of a regression problem using a k-Nearest Neighbor and the interpolation of the target using both barycenter and constant weights.
# Authors: The scikit-learn developers
# SPDX-License-Identifier: BSD-3-Clause
Generate sample data#
Here we generate a few data points to use to train the model. We also generate data in the whole range of the training data to visualize how the model would react in that whole region.
import matplotlib.pyplot as plt
import numpy as np
from sklearn import neighbors
rng = np.random.RandomState(0)
X_train = np.sort(5 * rng.rand(40, 1), axis=0)
X_test = np.linspace(0, 5, 500)[:, np.newaxis]
y = np.sin(X_train).ravel()
# Add noise to targets
y[::5] += 1 * (0.5 - np.random.rand(8))
Fit regression model#
Here we train a model and visualize how uniform
and distance
weights in prediction effect predicted values.
n_neighbors = 5
for i, weights in enumerate(["uniform", "distance"]):
knn = neighbors.KNeighborsRegressor(n_neighbors, weights=weights)
y_ = knn.fit(X_train, y).predict(X_test)
plt.subplot(2, 1, i + 1)
plt.scatter(X_train, y, color="darkorange", label="data")
plt.plot(X_test, y_, color="navy", label="prediction")
plt.axis("tight")
plt.legend()
plt.title("KNeighborsRegressor (k = %i, weights = '%s')" % (n_neighbors, weights))
plt.tight_layout()
plt.show()
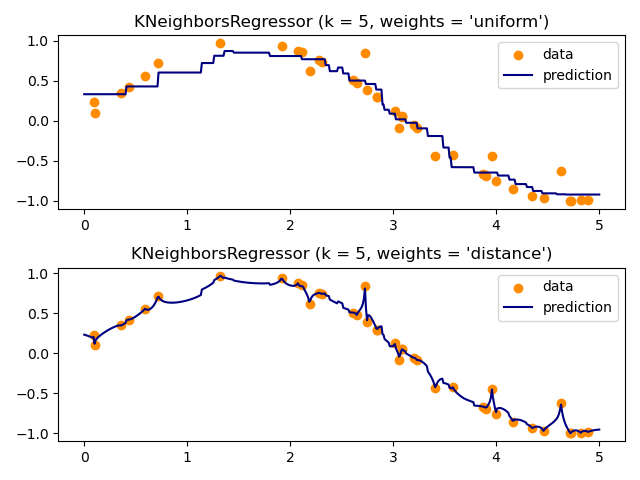
Total running time of the script: (0 minutes 0.246 seconds)
Related examples
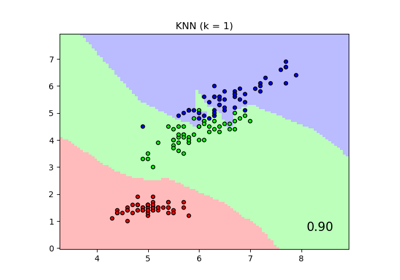
Comparing Nearest Neighbors with and without Neighborhood Components Analysis
Comparing Nearest Neighbors with and without Neighborhood Components Analysis