Note
Go to the end to download the full example code. or to run this example in your browser via JupyterLite or Binder
Plot Hierarchical Clustering Dendrogram#
This example plots the corresponding dendrogram of a hierarchical clustering using AgglomerativeClustering and the dendrogram method available in scipy.
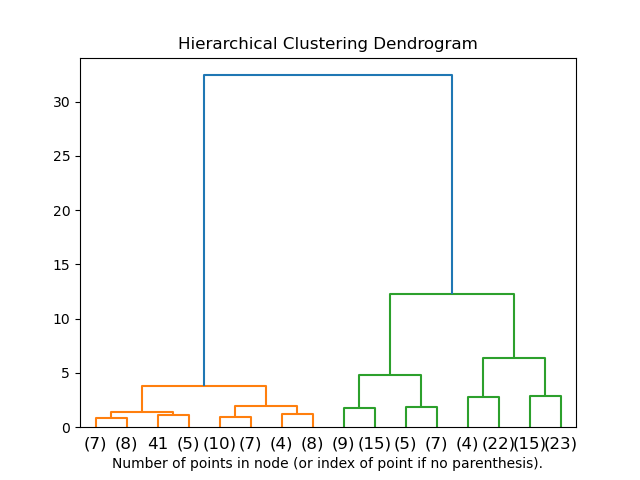
import numpy as np
from matplotlib import pyplot as plt
from scipy.cluster.hierarchy import dendrogram
from sklearn.cluster import AgglomerativeClustering
from sklearn.datasets import load_iris
def plot_dendrogram(model, **kwargs):
# Create linkage matrix and then plot the dendrogram
# create the counts of samples under each node
counts = np.zeros(model.children_.shape[0])
n_samples = len(model.labels_)
for i, merge in enumerate(model.children_):
current_count = 0
for child_idx in merge:
if child_idx < n_samples:
current_count += 1 # leaf node
else:
current_count += counts[child_idx - n_samples]
counts[i] = current_count
linkage_matrix = np.column_stack(
[model.children_, model.distances_, counts]
).astype(float)
# Plot the corresponding dendrogram
dendrogram(linkage_matrix, **kwargs)
iris = load_iris()
X = iris.data
# setting distance_threshold=0 ensures we compute the full tree.
model = AgglomerativeClustering(distance_threshold=0, n_clusters=None)
model = model.fit(X)
plt.title("Hierarchical Clustering Dendrogram")
# plot the top three levels of the dendrogram
plot_dendrogram(model, truncate_mode="level", p=3)
plt.xlabel("Number of points in node (or index of point if no parenthesis).")
plt.show()
Total running time of the script: (0 minutes 0.096 seconds)
Related examples
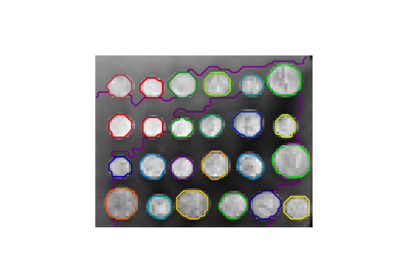
A demo of structured Ward hierarchical clustering on an image of coins
A demo of structured Ward hierarchical clustering on an image of coins
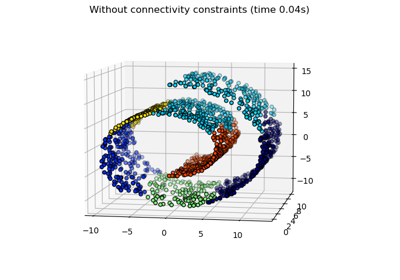
Hierarchical clustering: structured vs unstructured ward
Hierarchical clustering: structured vs unstructured ward
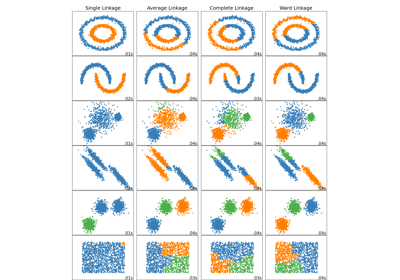
Comparing different hierarchical linkage methods on toy datasets
Comparing different hierarchical linkage methods on toy datasets