sklearn.pipeline
.make_pipeline¶
- sklearn.pipeline.make_pipeline(*steps, memory=None, verbose=False)[source]¶
Construct a
Pipeline
from the given estimators.This is a shorthand for the
Pipeline
constructor; it does not require, and does not permit, naming the estimators. Instead, their names will be set to the lowercase of their types automatically.- Parameters:
- *stepslist of Estimator objects
List of the scikit-learn estimators that are chained together.
- memorystr or object with the joblib.Memory interface, default=None
Used to cache the fitted transformers of the pipeline. The last step will never be cached, even if it is a transformer. By default, no caching is performed. If a string is given, it is the path to the caching directory. Enabling caching triggers a clone of the transformers before fitting. Therefore, the transformer instance given to the pipeline cannot be inspected directly. Use the attribute
named_steps
orsteps
to inspect estimators within the pipeline. Caching the transformers is advantageous when fitting is time consuming.- verbosebool, default=False
If True, the time elapsed while fitting each step will be printed as it is completed.
- Returns:
- pPipeline
Returns a scikit-learn
Pipeline
object.
See also
Pipeline
Class for creating a pipeline of transforms with a final estimator.
Examples
>>> from sklearn.naive_bayes import GaussianNB >>> from sklearn.preprocessing import StandardScaler >>> from sklearn.pipeline import make_pipeline >>> make_pipeline(StandardScaler(), GaussianNB(priors=None)) Pipeline(steps=[('standardscaler', StandardScaler()), ('gaussiannb', GaussianNB())])
Examples using sklearn.pipeline.make_pipeline
¶
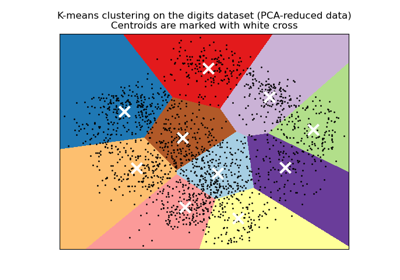
A demo of K-Means clustering on the handwritten digits data
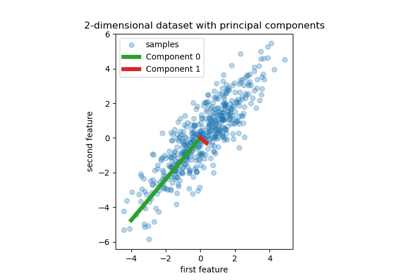
Principal Component Regression vs Partial Least Squares Regression
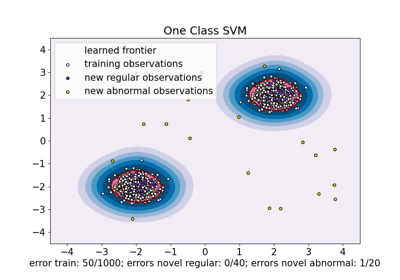
One-Class SVM versus One-Class SVM using Stochastic Gradient Descent
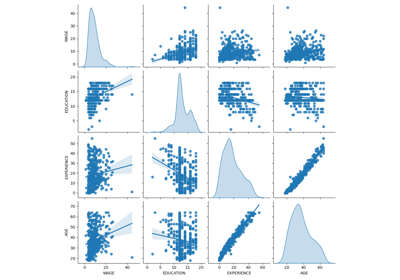
Common pitfalls in the interpretation of coefficients of linear models
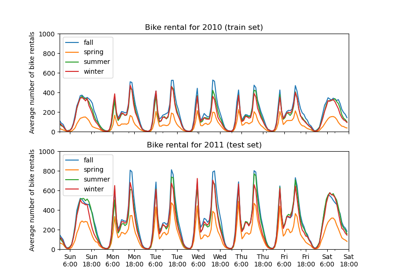
Partial Dependence and Individual Conditional Expectation Plots
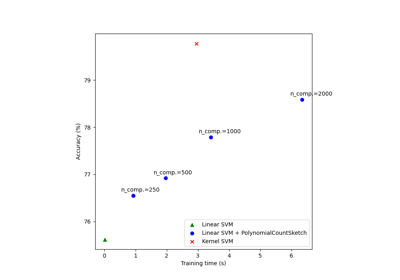
Scalable learning with polynomial kernel approximation
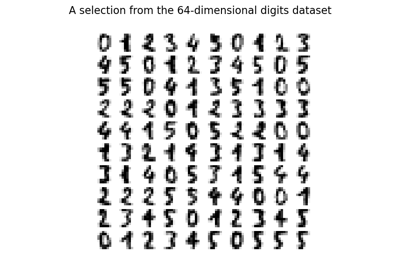
Manifold learning on handwritten digits: Locally Linear Embedding, Isomap…
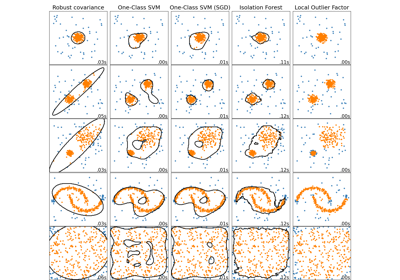
Comparing anomaly detection algorithms for outlier detection on toy datasets
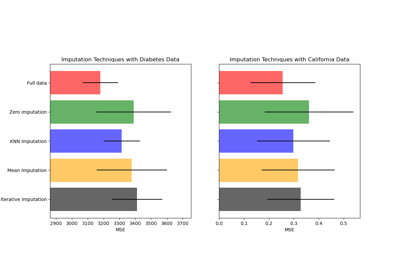
Imputing missing values before building an estimator
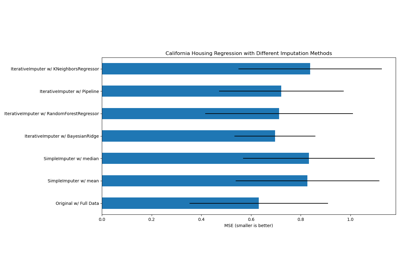
Imputing missing values with variants of IterativeImputer
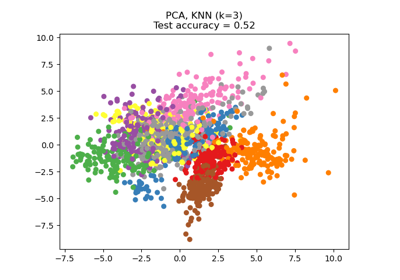
Dimensionality Reduction with Neighborhood Components Analysis