This is documentation for an old release of Scikit-learn (version 0.22). Try the latest stable release (version 1.6) or development (unstable) versions.
Note
Click here to download the full example code or to run this example in your browser via Binder
SVM Tie Breaking Example¶
Tie breaking is costly if decision_function_shape='ovr'
, and therefore it
is not enabled by default. This example illustrates the effect of the
break_ties
parameter for a multiclass classification problem and
decision_function_shape='ovr'
.
The two plots differ only in the area in the middle where the classes are
tied. If break_ties=False
, all input in that area would be classified as
one class, whereas if break_ties=True
, the tie-breaking mechanism will
create a non-convex decision boundary in that area.
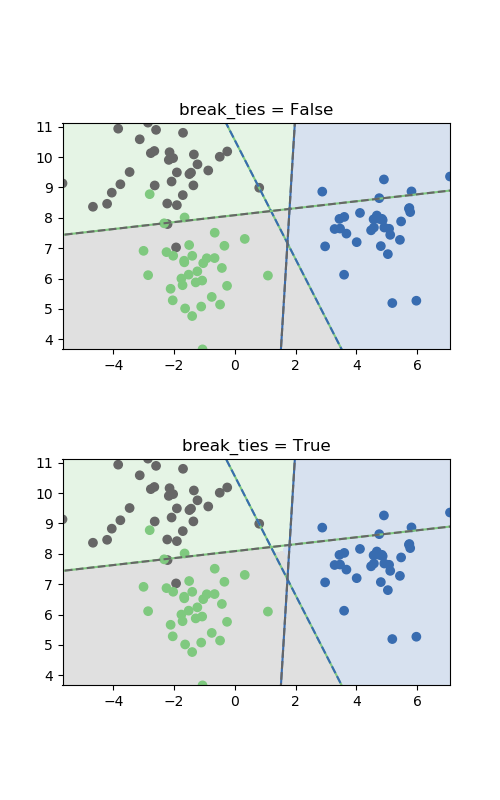
print(__doc__)
# Code source: Andreas Mueller, Adrin Jalali
# License: BSD 3 clause
import numpy as np
import matplotlib.pyplot as plt
from sklearn.svm import SVC
from sklearn.datasets import make_blobs
X, y = make_blobs(random_state=27)
fig, sub = plt.subplots(2, 1, figsize=(5, 8))
titles = ("break_ties = False",
"break_ties = True")
for break_ties, title, ax in zip((False, True), titles, sub.flatten()):
svm = SVC(kernel="linear", C=1, break_ties=break_ties,
decision_function_shape='ovr').fit(X, y)
xlim = [X[:, 0].min(), X[:, 0].max()]
ylim = [X[:, 1].min(), X[:, 1].max()]
xs = np.linspace(xlim[0], xlim[1], 1000)
ys = np.linspace(ylim[0], ylim[1], 1000)
xx, yy = np.meshgrid(xs, ys)
pred = svm.predict(np.c_[xx.ravel(), yy.ravel()])
colors = [plt.cm.Accent(i) for i in [0, 4, 7]]
points = ax.scatter(X[:, 0], X[:, 1], c=y, cmap="Accent")
classes = [(0, 1), (0, 2), (1, 2)]
line = np.linspace(X[:, 1].min() - 5, X[:, 1].max() + 5)
ax.imshow(-pred.reshape(xx.shape), cmap="Accent", alpha=.2,
extent=(xlim[0], xlim[1], ylim[1], ylim[0]))
for coef, intercept, col in zip(svm.coef_, svm.intercept_, classes):
line2 = -(line * coef[1] + intercept) / coef[0]
ax.plot(line2, line, "-", c=colors[col[0]])
ax.plot(line2, line, "--", c=colors[col[1]])
ax.set_xlim(xlim)
ax.set_ylim(ylim)
ax.set_title(title)
ax.set_aspect("equal")
plt.show()
Total running time of the script: ( 0 minutes 1.207 seconds)
Estimated memory usage: 86 MB