This is documentation for an old release of Scikit-learn (version 0.22). Try the latest stable release (version 1.6) or development (unstable) versions.
Note
Click here to download the full example code or to run this example in your browser via Binder
Gaussian process classification (GPC) on iris dataset¶
This example illustrates the predicted probability of GPC for an isotropic and anisotropic RBF kernel on a two-dimensional version for the iris-dataset. The anisotropic RBF kernel obtains slightly higher log-marginal-likelihood by assigning different length-scales to the two feature dimensions.
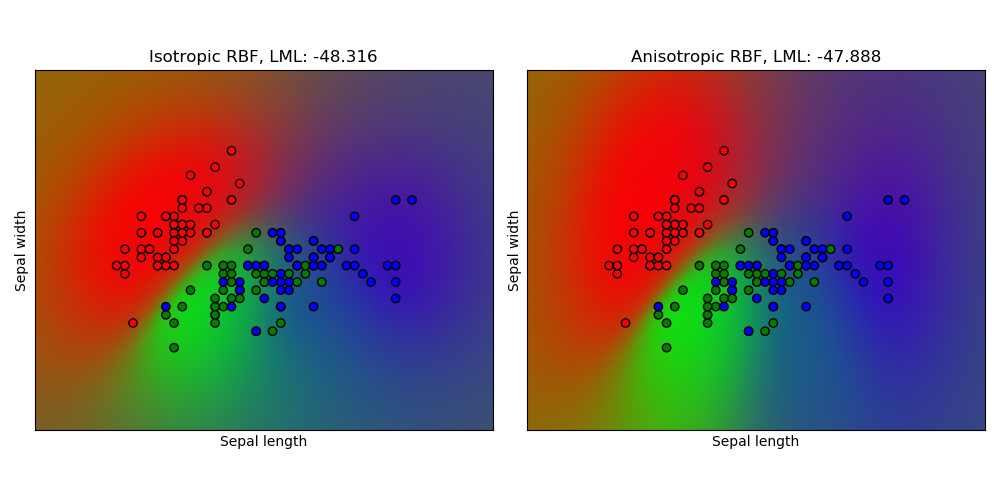
print(__doc__)
import numpy as np
import matplotlib.pyplot as plt
from sklearn import datasets
from sklearn.gaussian_process import GaussianProcessClassifier
from sklearn.gaussian_process.kernels import RBF
# import some data to play with
iris = datasets.load_iris()
X = iris.data[:, :2] # we only take the first two features.
y = np.array(iris.target, dtype=int)
h = .02 # step size in the mesh
kernel = 1.0 * RBF([1.0])
gpc_rbf_isotropic = GaussianProcessClassifier(kernel=kernel).fit(X, y)
kernel = 1.0 * RBF([1.0, 1.0])
gpc_rbf_anisotropic = GaussianProcessClassifier(kernel=kernel).fit(X, y)
# create a mesh to plot in
x_min, x_max = X[:, 0].min() - 1, X[:, 0].max() + 1
y_min, y_max = X[:, 1].min() - 1, X[:, 1].max() + 1
xx, yy = np.meshgrid(np.arange(x_min, x_max, h),
np.arange(y_min, y_max, h))
titles = ["Isotropic RBF", "Anisotropic RBF"]
plt.figure(figsize=(10, 5))
for i, clf in enumerate((gpc_rbf_isotropic, gpc_rbf_anisotropic)):
# Plot the predicted probabilities. For that, we will assign a color to
# each point in the mesh [x_min, m_max]x[y_min, y_max].
plt.subplot(1, 2, i + 1)
Z = clf.predict_proba(np.c_[xx.ravel(), yy.ravel()])
# Put the result into a color plot
Z = Z.reshape((xx.shape[0], xx.shape[1], 3))
plt.imshow(Z, extent=(x_min, x_max, y_min, y_max), origin="lower")
# Plot also the training points
plt.scatter(X[:, 0], X[:, 1], c=np.array(["r", "g", "b"])[y],
edgecolors=(0, 0, 0))
plt.xlabel('Sepal length')
plt.ylabel('Sepal width')
plt.xlim(xx.min(), xx.max())
plt.ylim(yy.min(), yy.max())
plt.xticks(())
plt.yticks(())
plt.title("%s, LML: %.3f" %
(titles[i], clf.log_marginal_likelihood(clf.kernel_.theta)))
plt.tight_layout()
plt.show()
Total running time of the script: ( 0 minutes 3.233 seconds)
Estimated memory usage: 286 MB