Note
Click here to download the full example code or to run this example in your browser via Binder
Feature agglomeration¶
These images how similar features are merged together using feature agglomeration.
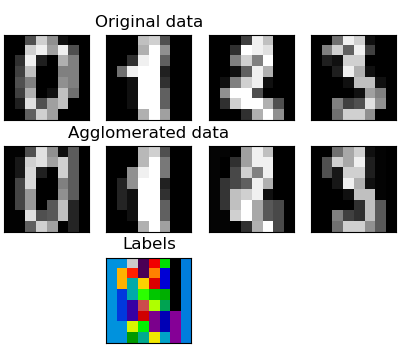
# Code source: Gaël Varoquaux
# Modified for documentation by Jaques Grobler
# License: BSD 3 clause
import numpy as np
import matplotlib.pyplot as plt
from sklearn import datasets, cluster
from sklearn.feature_extraction.image import grid_to_graph
digits = datasets.load_digits()
images = digits.images
X = np.reshape(images, (len(images), -1))
connectivity = grid_to_graph(*images[0].shape)
agglo = cluster.FeatureAgglomeration(connectivity=connectivity, n_clusters=32)
agglo.fit(X)
X_reduced = agglo.transform(X)
X_restored = agglo.inverse_transform(X_reduced)
images_restored = np.reshape(X_restored, images.shape)
plt.figure(1, figsize=(4, 3.5))
plt.clf()
plt.subplots_adjust(left=0.01, right=0.99, bottom=0.01, top=0.91)
for i in range(4):
plt.subplot(3, 4, i + 1)
plt.imshow(images[i], cmap=plt.cm.gray, vmax=16, interpolation="nearest")
plt.xticks(())
plt.yticks(())
if i == 1:
plt.title("Original data")
plt.subplot(3, 4, 4 + i + 1)
plt.imshow(images_restored[i], cmap=plt.cm.gray, vmax=16, interpolation="nearest")
if i == 1:
plt.title("Agglomerated data")
plt.xticks(())
plt.yticks(())
plt.subplot(3, 4, 10)
plt.imshow(
np.reshape(agglo.labels_, images[0].shape),
interpolation="nearest",
cmap=plt.cm.nipy_spectral,
)
plt.xticks(())
plt.yticks(())
plt.title("Labels")
plt.show()
Total running time of the script: ( 0 minutes 0.156 seconds)