Note
Click here to download the full example code or to run this example in your browser via Binder
Normal and Shrinkage Linear Discriminant Analysis for classification¶
Shows how shrinkage improves classification.
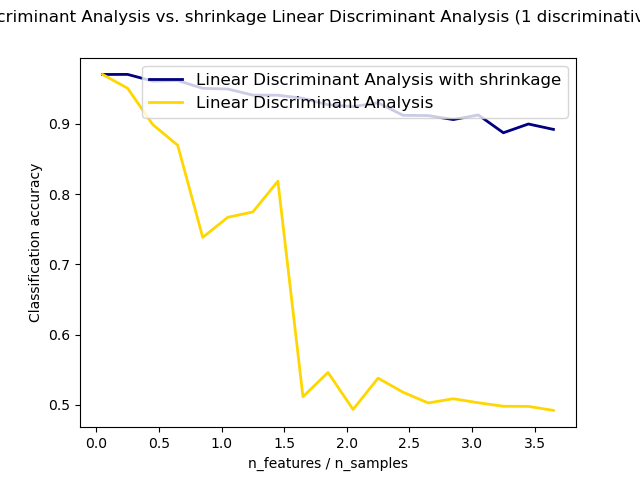
import numpy as np
import matplotlib.pyplot as plt
from sklearn.datasets import make_blobs
from sklearn.discriminant_analysis import LinearDiscriminantAnalysis
n_train = 20 # samples for training
n_test = 200 # samples for testing
n_averages = 50 # how often to repeat classification
n_features_max = 75 # maximum number of features
step = 4 # step size for the calculation
def generate_data(n_samples, n_features):
"""Generate random blob-ish data with noisy features.
This returns an array of input data with shape `(n_samples, n_features)`
and an array of `n_samples` target labels.
Only one feature contains discriminative information, the other features
contain only noise.
"""
X, y = make_blobs(n_samples=n_samples, n_features=1, centers=[[-2], [2]])
# add non-discriminative features
if n_features > 1:
X = np.hstack([X, np.random.randn(n_samples, n_features - 1)])
return X, y
acc_clf1, acc_clf2 = [], []
n_features_range = range(1, n_features_max + 1, step)
for n_features in n_features_range:
score_clf1, score_clf2 = 0, 0
for _ in range(n_averages):
X, y = generate_data(n_train, n_features)
clf1 = LinearDiscriminantAnalysis(solver='lsqr', shrinkage='auto').fit(X, y)
clf2 = LinearDiscriminantAnalysis(solver='lsqr', shrinkage=None).fit(X, y)
X, y = generate_data(n_test, n_features)
score_clf1 += clf1.score(X, y)
score_clf2 += clf2.score(X, y)
acc_clf1.append(score_clf1 / n_averages)
acc_clf2.append(score_clf2 / n_averages)
features_samples_ratio = np.array(n_features_range) / n_train
plt.plot(features_samples_ratio, acc_clf1, linewidth=2,
label="Linear Discriminant Analysis with shrinkage", color='navy')
plt.plot(features_samples_ratio, acc_clf2, linewidth=2,
label="Linear Discriminant Analysis", color='gold')
plt.xlabel('n_features / n_samples')
plt.ylabel('Classification accuracy')
plt.legend(loc=1, prop={'size': 12})
plt.suptitle('Linear Discriminant Analysis vs. \
shrinkage Linear Discriminant Analysis (1 discriminative feature)')
plt.show()
Total running time of the script: ( 0 minutes 3.883 seconds)