Note
Go to the end to download the full example code or to run this example in your browser via JupyterLite or Binder
Overview of multiclass training meta-estimators¶
In this example, we discuss the problem of classification when the target variable is composed of more than two classes. This is called multiclass classification.
In scikit-learn, all estimators support multiclass classification out of the
box: the most sensible strategy was implemented for the end-user. The
sklearn.multiclass
module implements various strategies that one can use
for experimenting or developing third-party estimators that only support binary
classification.
sklearn.multiclass
includes OvO/OvR strategies used to train a
multiclass classifier by fitting a set of binary classifiers (the
OneVsOneClassifier
and
OneVsRestClassifier
meta-estimators). This example
will review them.
The Yeast UCI dataset¶
In this example, we use a UCI dataset [1], generally referred as the Yeast
dataset. We use the sklearn.datasets.fetch_openml
function to load
the dataset from OpenML.
from sklearn.datasets import fetch_openml
X, y = fetch_openml(data_id=181, as_frame=True, return_X_y=True)
To know the type of data science problem we are dealing with, we can check the target for which we want to build a predictive model.
y.value_counts().sort_index()
class_protein_localization
CYT 463
ERL 5
EXC 35
ME1 44
ME2 51
ME3 163
MIT 244
NUC 429
POX 20
VAC 30
Name: count, dtype: int64
We see that the target is discrete and composed of 10 classes. We therefore deal with a multiclass classification problem.
Strategies comparison¶
In the following experiment, we use a
DecisionTreeClassifier
and a
RepeatedStratifiedKFold
cross-validation
with 3 splits and 5 repetitions.
We compare the following strategies:
:class:~sklearn.tree.DecisionTreeClassifier can handle multiclass classification without needing any special adjustments. It works by breaking down the training data into smaller subsets and focusing on the most common class in each subset. By repeating this process, the model can accurately classify input data into multiple different classes.
OneVsOneClassifier
trains a set of binary classifiers where each classifier is trained to distinguish between two classes.OneVsRestClassifier
: trains a set of binary classifiers where each classifier is trained to distinguish between one class and the rest of the classes.OutputCodeClassifier
: trains a set of binary classifiers where each classifier is trained to distinguish between a set of classes from the rest of the classes. The set of classes is defined by a codebook, which is randomly generated in scikit-learn. This method exposes a parametercode_size
to control the size of the codebook. We set it above one since we are not interested in compressing the class representation.
import pandas as pd
from sklearn.model_selection import RepeatedStratifiedKFold, cross_validate
from sklearn.multiclass import (
OneVsOneClassifier,
OneVsRestClassifier,
OutputCodeClassifier,
)
from sklearn.tree import DecisionTreeClassifier
cv = RepeatedStratifiedKFold(n_splits=3, n_repeats=5, random_state=0)
tree = DecisionTreeClassifier(random_state=0)
ovo_tree = OneVsOneClassifier(tree)
ovr_tree = OneVsRestClassifier(tree)
ecoc = OutputCodeClassifier(tree, code_size=2)
cv_results_tree = cross_validate(tree, X, y, cv=cv, n_jobs=2)
cv_results_ovo = cross_validate(ovo_tree, X, y, cv=cv, n_jobs=2)
cv_results_ovr = cross_validate(ovr_tree, X, y, cv=cv, n_jobs=2)
cv_results_ecoc = cross_validate(ecoc, X, y, cv=cv, n_jobs=2)
We can now compare the statistical performance of the different strategies. We plot the score distribution of the different strategies.
from matplotlib import pyplot as plt
scores = pd.DataFrame(
{
"DecisionTreeClassifier": cv_results_tree["test_score"],
"OneVsOneClassifier": cv_results_ovo["test_score"],
"OneVsRestClassifier": cv_results_ovr["test_score"],
"OutputCodeClassifier": cv_results_ecoc["test_score"],
}
)
ax = scores.plot.kde(legend=True)
ax.set_xlabel("Accuracy score")
ax.set_xlim([0, 0.7])
_ = ax.set_title(
"Density of the accuracy scores for the different multiclass strategies"
)
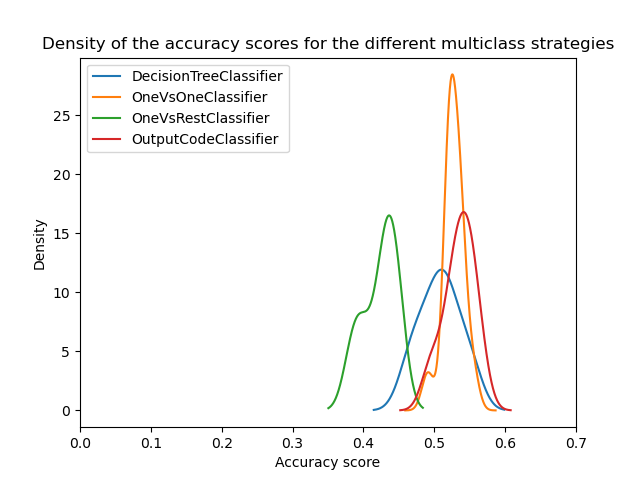
At a first glance, we can see that the built-in strategy of the decision tree classifier is working quite well. One-vs-one and the error-correcting output code strategies are working even better. However, the one-vs-rest strategy is not working as well as the other strategies.
Indeed, these results reproduce something reported in the literature as in [2]. However, the story is not as simple as it seems.
The importance of hyperparameters search¶
It was later shown in [3] that the multiclass strategies would show similar scores if the hyperparameters of the base classifiers are first optimized.
Here we try to reproduce such result by at least optimizing the depth of the base decision tree.
from sklearn.model_selection import GridSearchCV
param_grid = {"max_depth": [3, 5, 8]}
tree_optimized = GridSearchCV(tree, param_grid=param_grid, cv=3)
ovo_tree = OneVsOneClassifier(tree_optimized)
ovr_tree = OneVsRestClassifier(tree_optimized)
ecoc = OutputCodeClassifier(tree_optimized, code_size=2)
cv_results_tree = cross_validate(tree_optimized, X, y, cv=cv, n_jobs=2)
cv_results_ovo = cross_validate(ovo_tree, X, y, cv=cv, n_jobs=2)
cv_results_ovr = cross_validate(ovr_tree, X, y, cv=cv, n_jobs=2)
cv_results_ecoc = cross_validate(ecoc, X, y, cv=cv, n_jobs=2)
scores = pd.DataFrame(
{
"DecisionTreeClassifier": cv_results_tree["test_score"],
"OneVsOneClassifier": cv_results_ovo["test_score"],
"OneVsRestClassifier": cv_results_ovr["test_score"],
"OutputCodeClassifier": cv_results_ecoc["test_score"],
}
)
ax = scores.plot.kde(legend=True)
ax.set_xlabel("Accuracy score")
ax.set_xlim([0, 0.7])
_ = ax.set_title(
"Density of the accuracy scores for the different multiclass strategies"
)
plt.show()
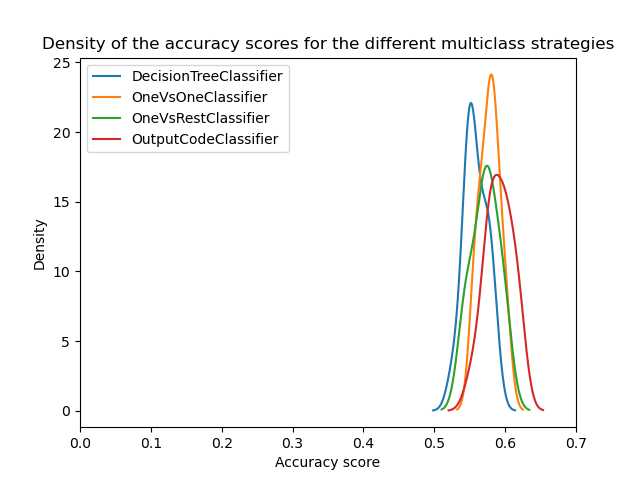
We can see that once the hyperparameters are optimized, all multiclass strategies have similar performance as discussed in [3].
Conclusion¶
We can get some intuition behind those results.
First, the reason for which one-vs-one and error-correcting output code are outperforming the tree when the hyperparameters are not optimized relies on fact that they ensemble a larger number of classifiers. The ensembling improves the generalization performance. This is a bit similar why a bagging classifier generally performs better than a single decision tree if no care is taken to optimize the hyperparameters.
Then, we see the importance of optimizing the hyperparameters. Indeed, it should be regularly explored when developing predictive models even if techniques such as ensembling help at reducing this impact.
Finally, it is important to recall that the estimators in scikit-learn are developed with a specific strategy to handle multiclass classification out of the box. So for these estimators, it means that there is no need to use different strategies. These strategies are mainly useful for third-party estimators supporting only binary classification. In all cases, we also show that the hyperparameters should be optimized.
References¶
Total running time of the script: (0 minutes 18.937 seconds)
Related examples
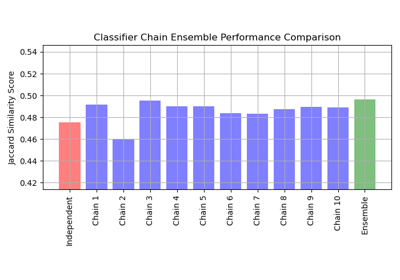
Multilabel classification using a classifier chain